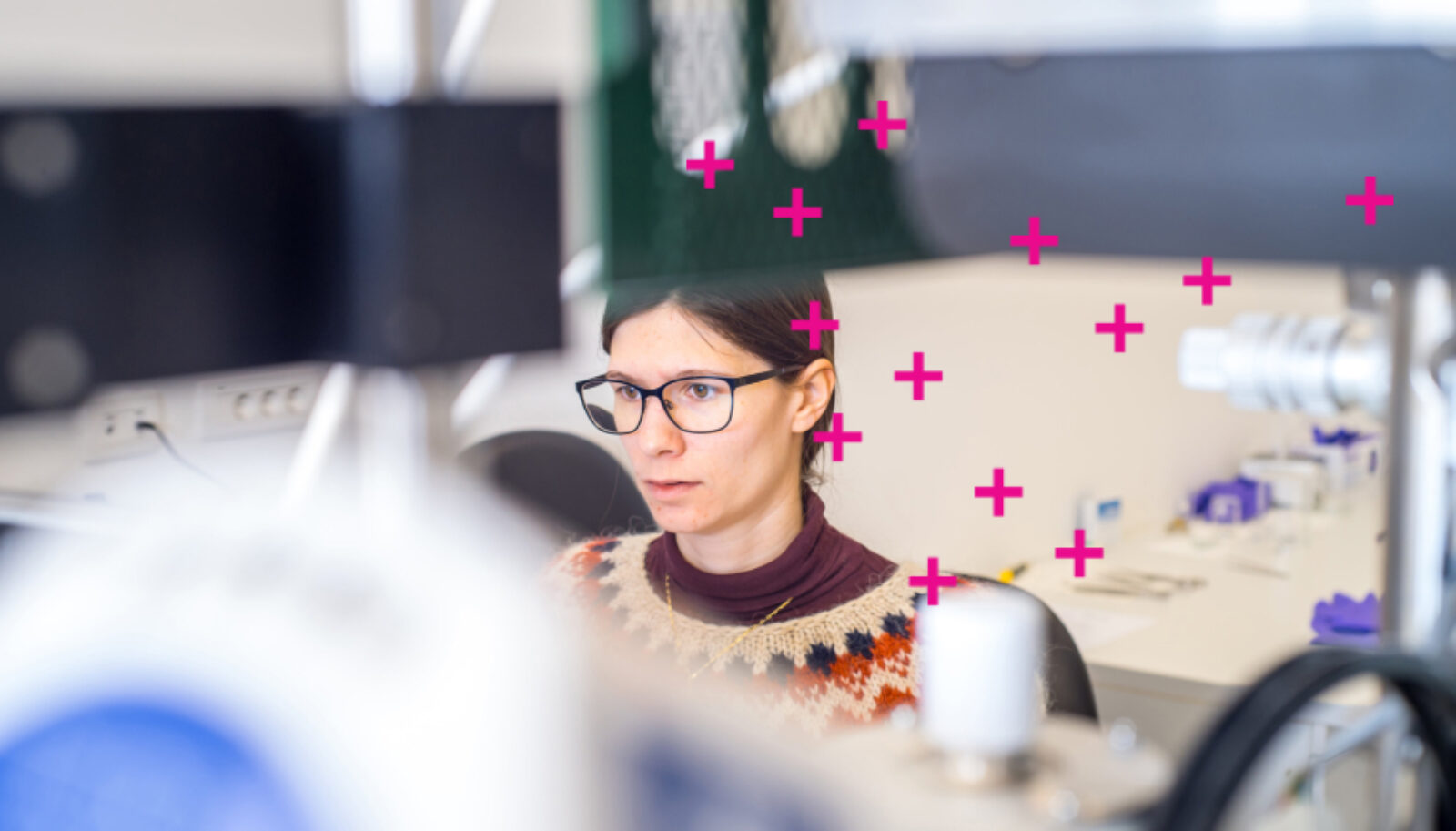
Vector attracts the world’s most accomplished and innovative AI and machine learning researchers
Our renowned research community is advancing breakthroughs in the science and application of AI. From using quantum computing to address climate change, to developing new machine learning models for 3D applications, harnessing AI to improve food price forecasting, and more, Vector researchers are unlocking new ways to apply AI to drive better economic, health, and societal outcomes.
Our strategic research priorities
- Machine Learning
- Deep Learning
- AI for Science
- Trustworthy AI
- AI for Health
- Foundation Models
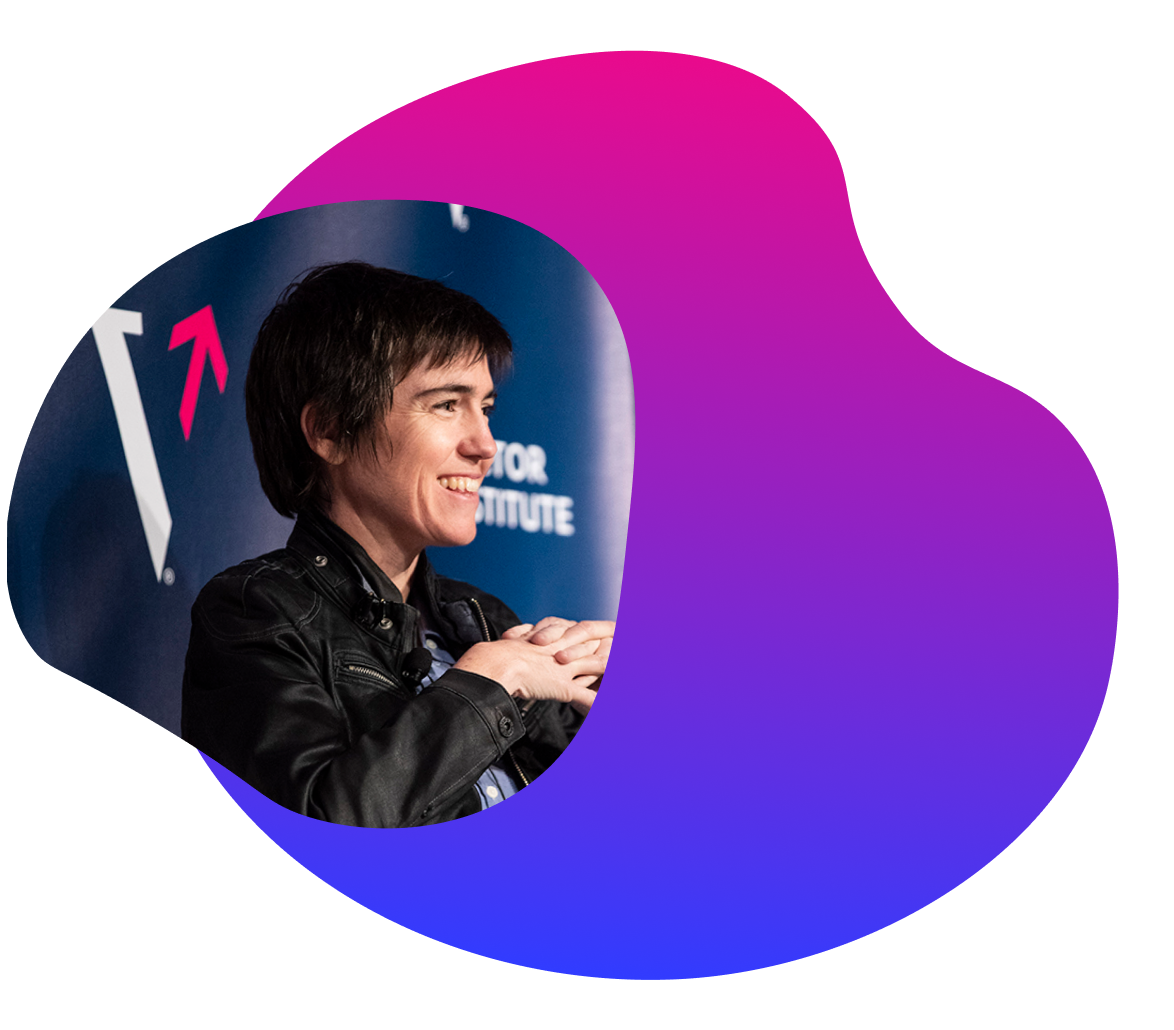
Vector is advancing its goal of becoming a top 10 global centre for AI research by attracting the world’s most accomplished, ambitious, and innovative researchers who are unlocking new achievements across a wide range of AI and machine learning topics.
714 Members of the Vector research community, comprising:
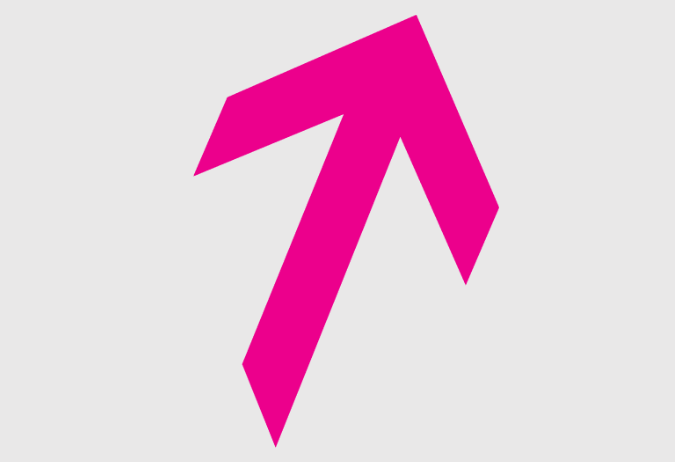
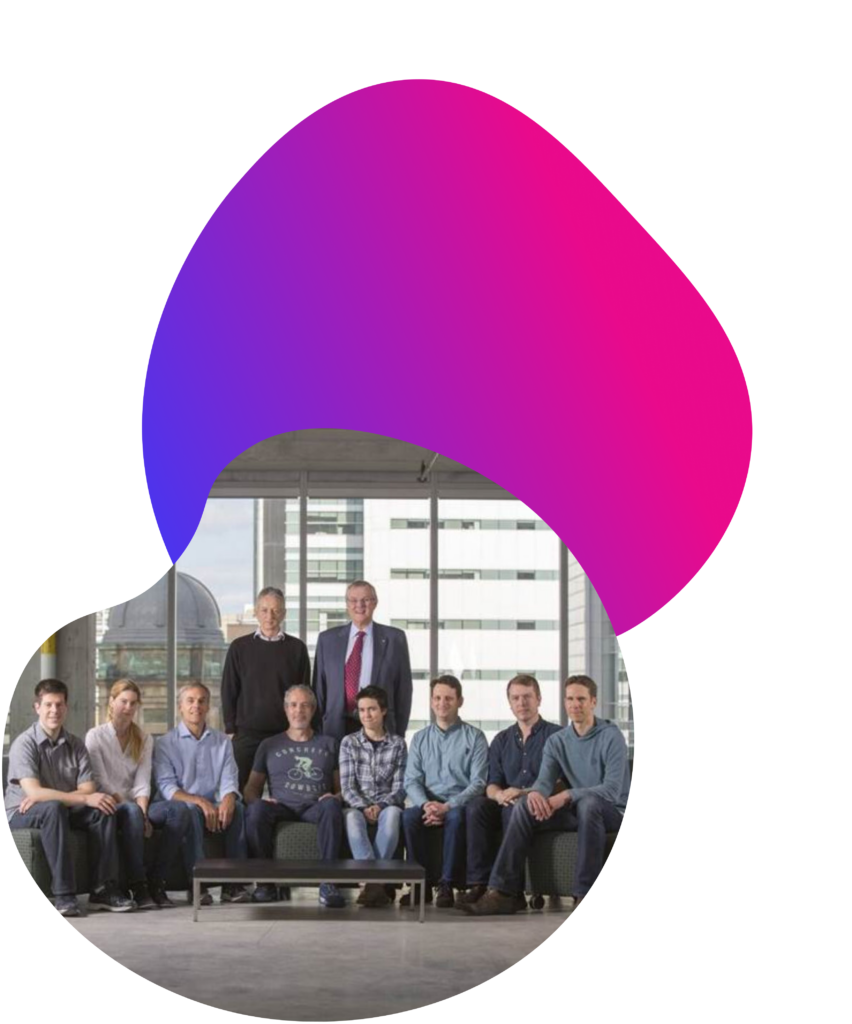
Our growing research team
What was once only a few founding faculty has evolved over the last five years into a flourishing community comprising over 700 researchers who are pushing the boundaries of AI, machine learning, and deep learning in critical areas to benefit Ontarians, Canadians, and people around the world.
We drive this growth through new and expanding efforts to attract and develop an outstanding community
- Fostering collaboration between industry and academia to connect leading research and AI applications.
- Creating more ways for researchers to work with industry sponsors and health sector partners on real-world problems and novel data sets.
- Expanding access to events focused on research and applications and increasing access to internships.
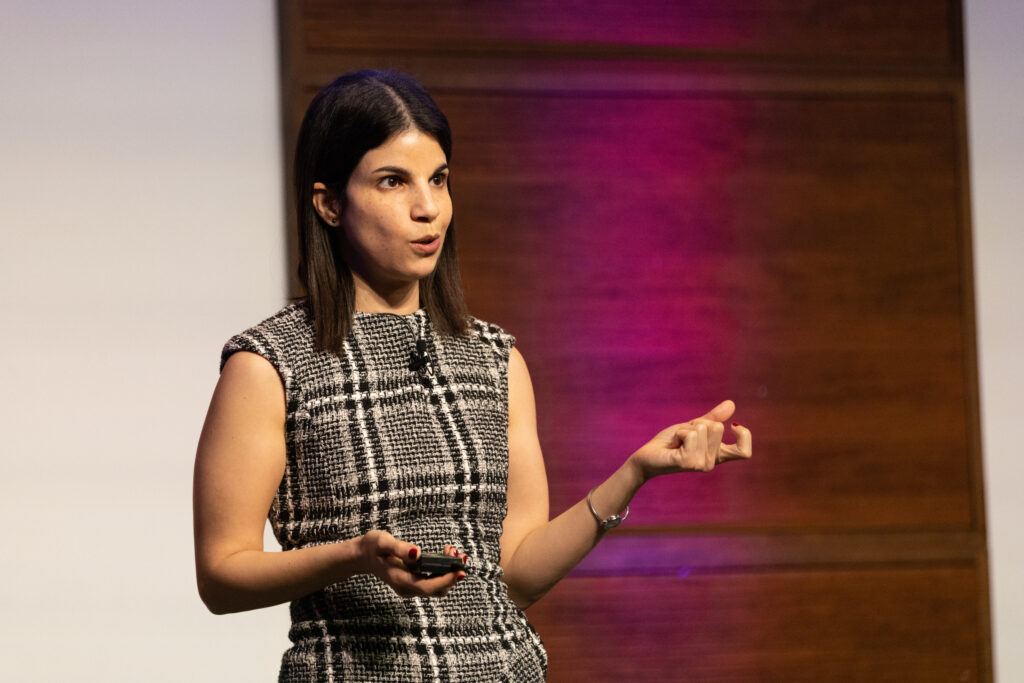
Published research
In dozens of timely, globally relevant, and impactful projects and work themes, these researchers are unlocking new ways to apply AI to drive better economic, health, and societal outcomes.
Latest research news
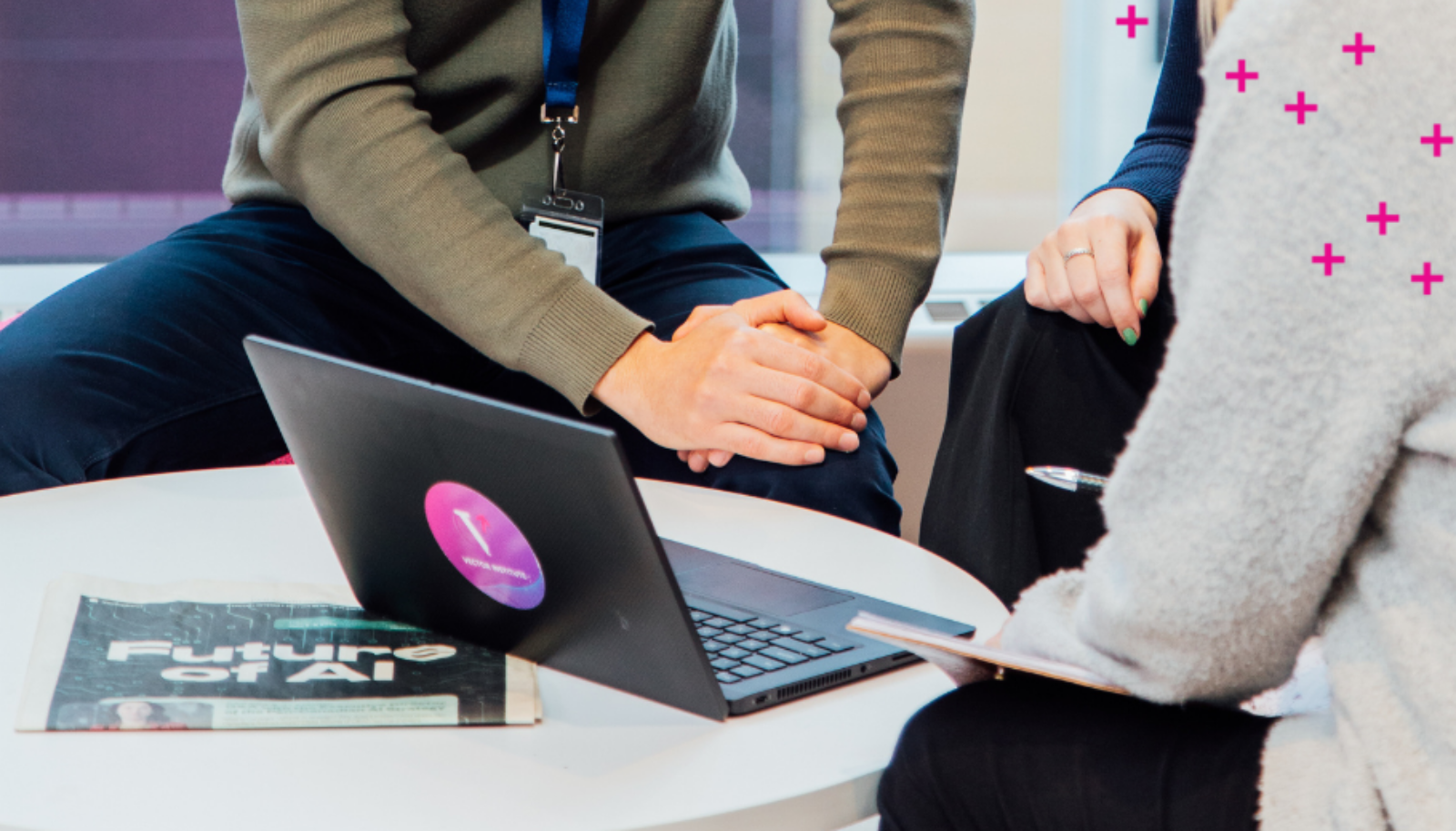
Benchmarking xAI’s Grok-1
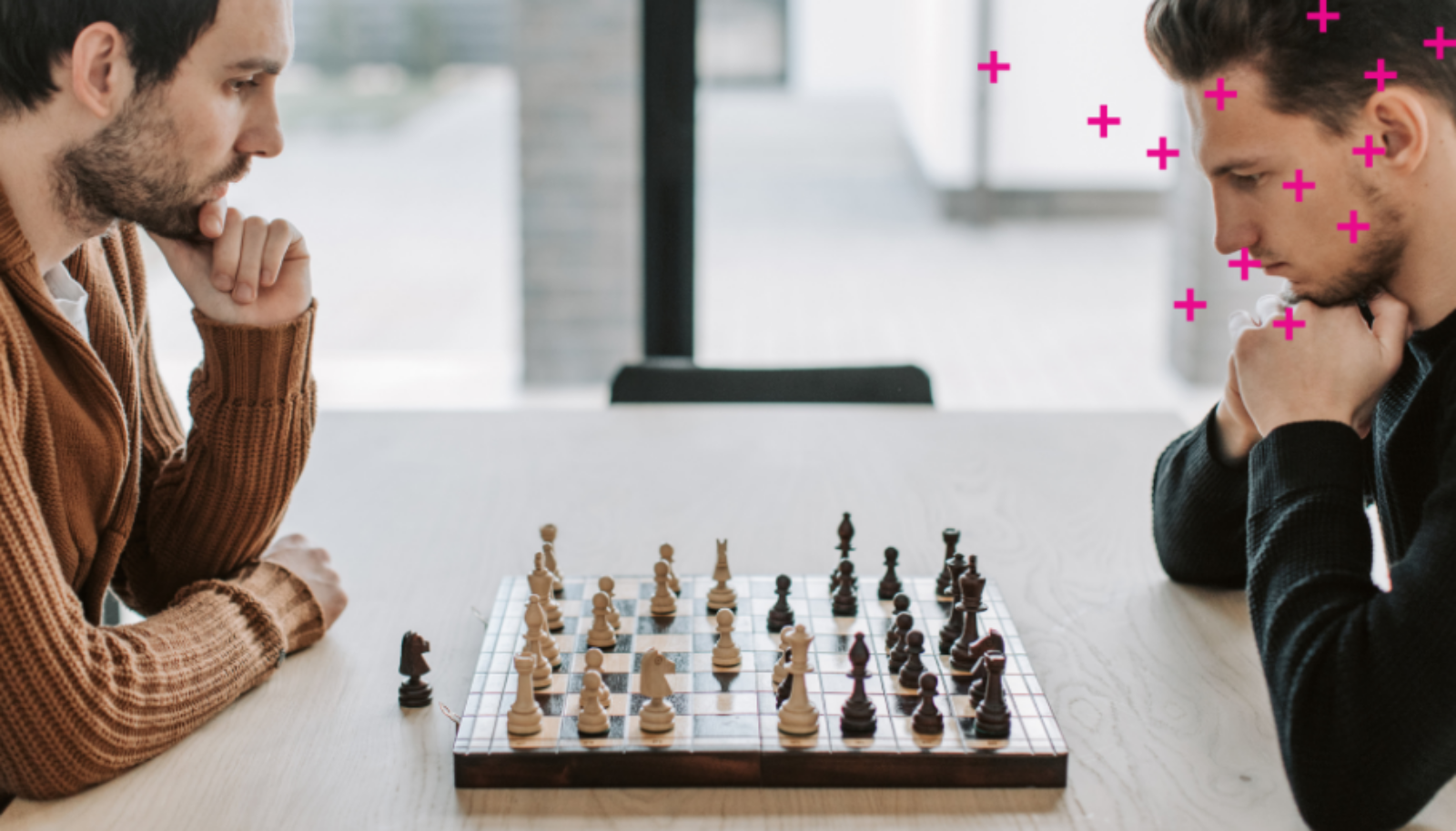
Vector Research Blog: Is Your Neural Network at Risk? The Pitfall of Adaptive Gradient Optimizers
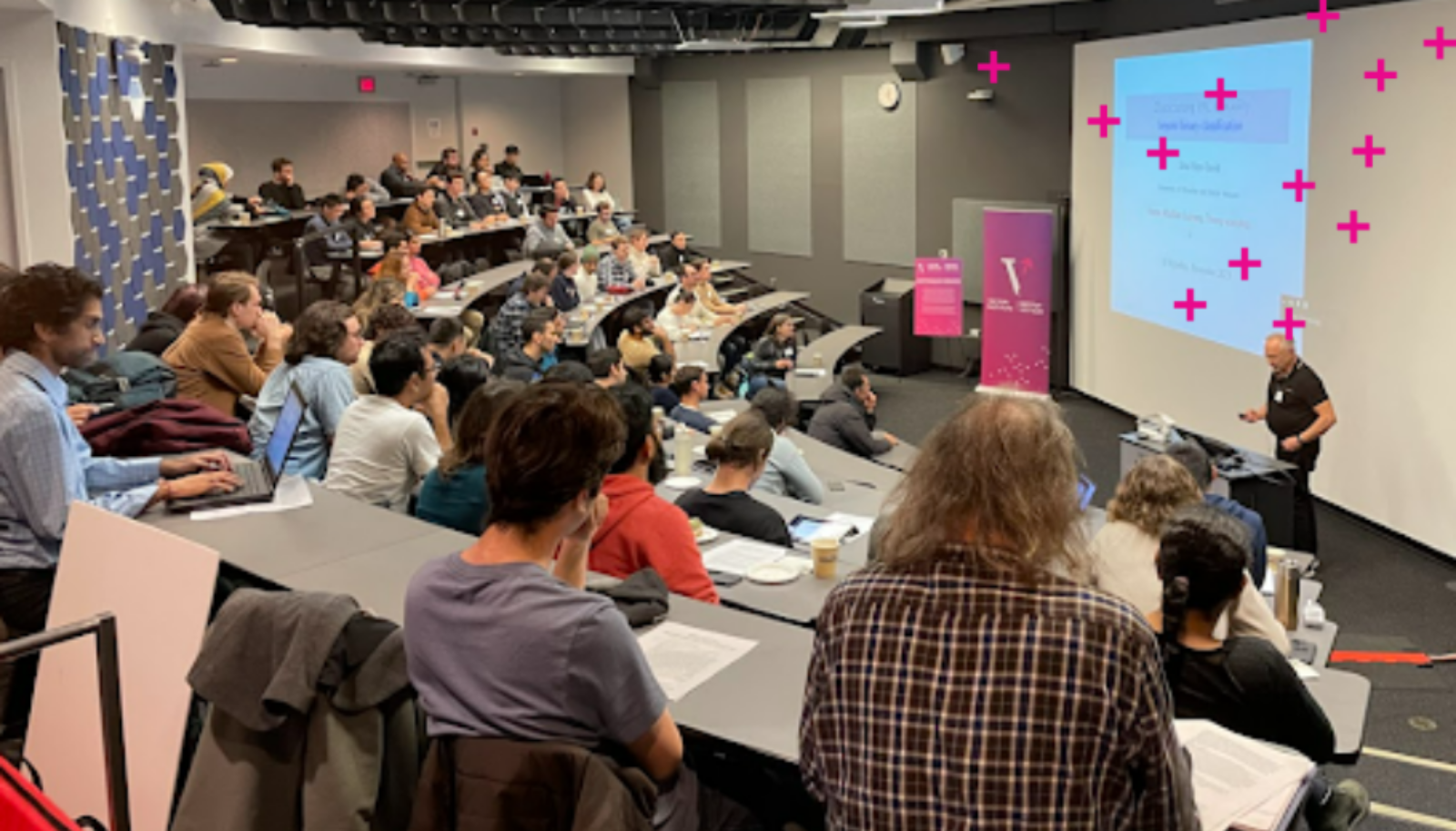
Machine learning theory takes centre stage at Vector Institute workshop
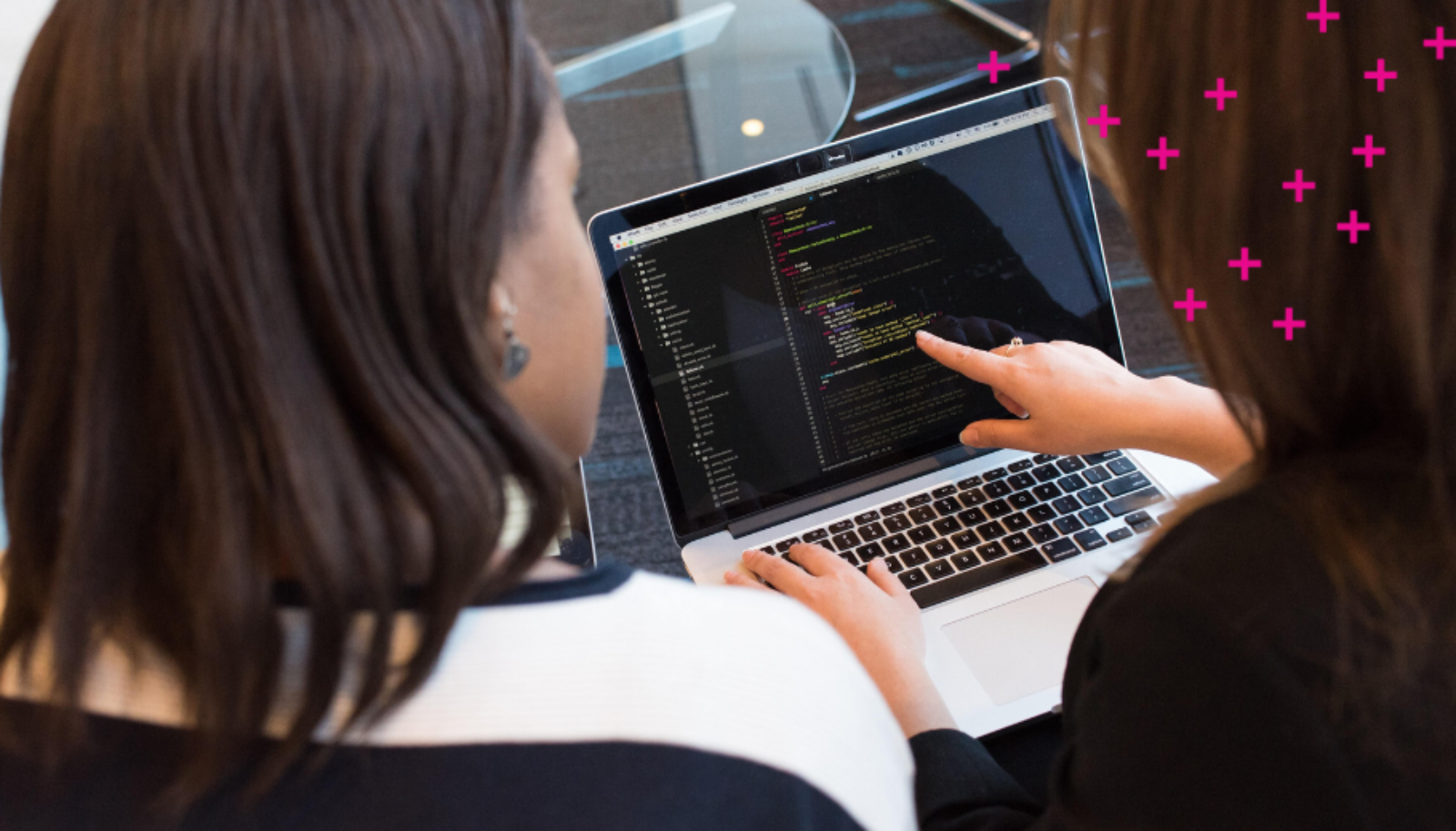
Introducing FlexModel: Breakthrough Framework for Unveiling the Secrets of Large Generative AI Models
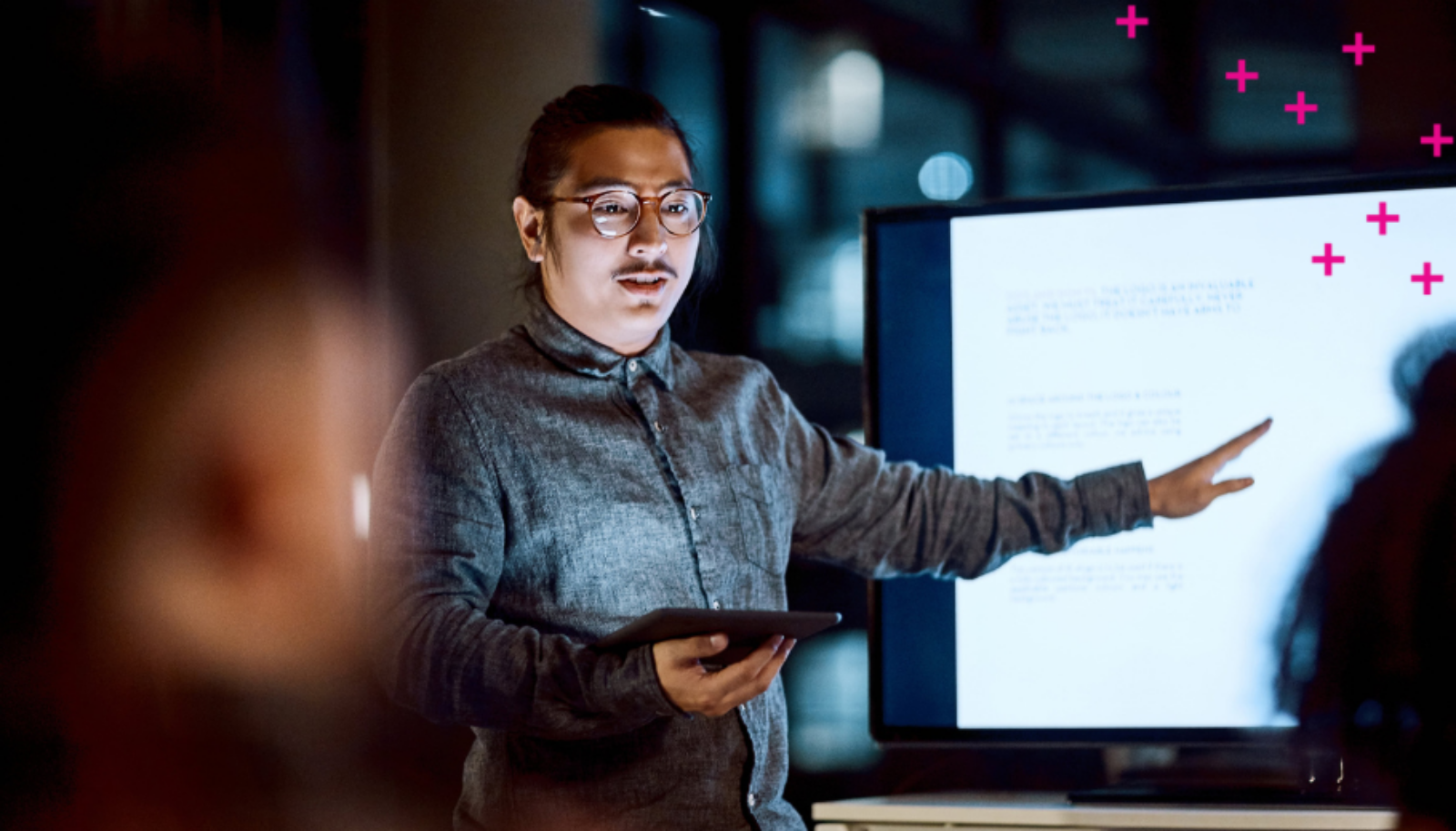
Neutralizing Bias in AI: Vector Institute’s UnBIAS Framework Revolutionizes Ethical Text Analysis
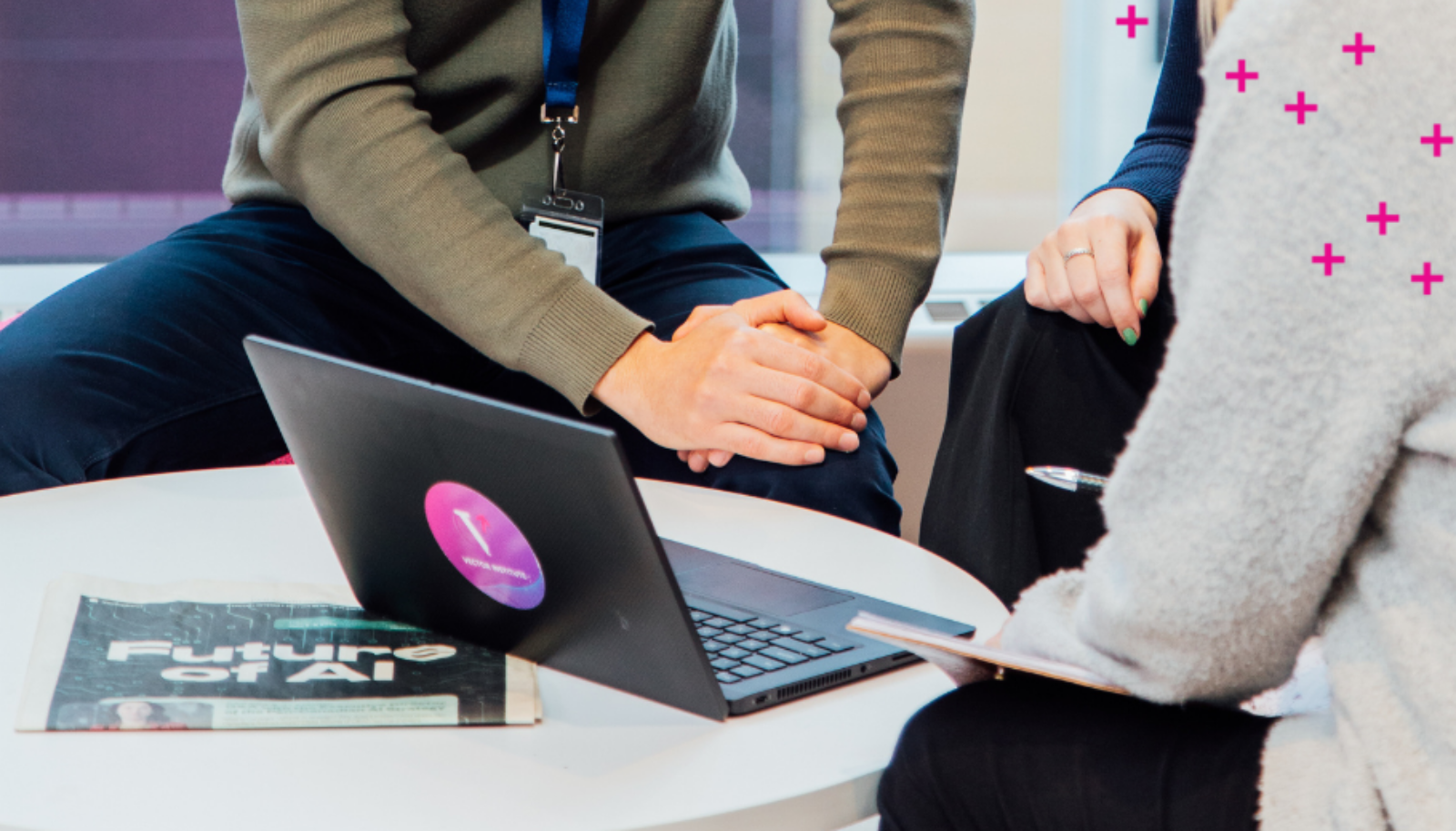
Vector researchers presenting more than 65 papers at NeurIPS 2023
Health Research
Improving health outcomes for everyone
Vector helps improve population health outcomes by creating an AI ecosystem that fosters innovation, enables better data collection and analysis, addresses staffing challenges, reduces wait times, and improves patient lives and care.
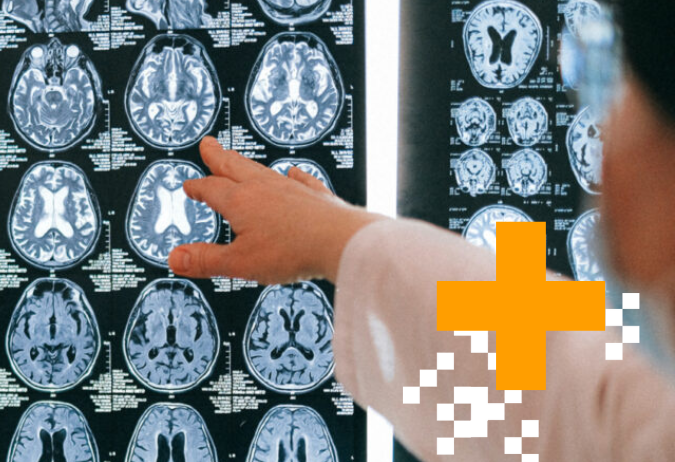
Upcoming Research Talks
Introducing the Vector Distinguished Lecture Series by the Vector Institute—a public talk series for academic and industrial data scientists in the GTA to discuss advanced machine learning topics.
Watch past talks
Promise and Pitfalls of Public Data in Private ML
Gautam Kamath, Vector Faculty Member
Troubling Trajectories for Uncertainty Quantification and Decision Making with Neural Networks
Geoff Pleiss, Vector Faculty Member
Build an Ecosystem, Not a Monolith
Colin Raffel, Vector Faculty Member
Graph Neural Networks meet Spectral Graph Theory: A Case Study
Renjie Liao, Vector Faculty Member
Random matrix theory for high dimensional optimization, and an application to scaling laws
Elliot Paquette, Associate Professor at McGill University
Keep up with the latest AI trends and research
Get all the latest AI news, advancements, and events straight into your inbox. Sign up for our monthly newsletter.